2021
SHREC 2021: Classification in cryo-electron tomograms
3D Object Retrieval Workshop 2021, Cardiff UK
Link: doi.org/10.2312/3dor.20211307
cryo-electron tomography simulator classification benchmark
Cryo-electron tomography (cryo-ET) is an imaging technique that allows three-dimensional visualization of macro-molecular assemblies under near-native conditions. Cryo-ET comes with a number of challenges, mainly low signal-to-noise and inability to obtain images from all angles. Computational methods are key to analyze cryo-electron tomograms. To promote innovation in computational methods, we generate a novel simulated dataset to benchmark different methods of localization and classification of biological macromolecules in tomograms. Our publicly available dataset contains ten tomographic reconstructions of simulated cell-like volumes. Each volume contains twelve different types of complexes, varying in size, function and structure. In this paper, we have evaluated seven different methods of finding and classifying proteins. Seven research groups present results obtained with learning-based methods and trained on the simulated dataset, as well as a baseline template matching (TM), a traditional method widely used in cryo-ET research. We show that learning-based approaches can achieve notably better localization and classification performance than TM. We also experimentally confirm that there is a negative relationship between particle size and performance for all methods.
2020
SHREC 2020: Classification in cryo-electron tomograms
3D Object Retrieval Workshop 2020, Genoa IT
Link: doi.org/10.1016/j.cag.2020.07.010
cryo-electron tomography simulator classification benchmark
Cryo-electron tomography (cryo-ET) is an imaging technique that allows us to three-dimensionally visualize both the structural details of macro-molecular assemblies under near-native conditions and its cellular context. Electrons strongly interact with biological samples, limiting electron dose. The latter limits the signal-to-noise ratio and hence resolution of an individual tomogram to about 50 Å (5 nm). Biological molecules can be obtained by averaging volumes, each depicting copies of the molecule, allowing for resolutions beyond 4 Å (0.4 nm). To this end, the ability to localize and classify components is crucial, but challenging due to the low signal-to-noise ratio. Computational innovation is key to mine biological information from cryo-electron tomography. To promote such innovation, we provide a novel simulated dataset to benchmark different methods of localization and classification of biological macromolecules in cryo-electron tomograms. Our publicly available dataset contains ten tomographic reconstructions of simulated cell-like volumes. Each volume contains twelve different types of complexes, varying in size, function and structure. In this paper, we have evaluated seven different methods of finding and classifying proteins. Six research groups present results obtained with learning-based methods and trained on the simulated dataset, as well as a baseline template matching, a traditional method widely used in cryo-ET research. We find that method performance correlates with particle size, especially noticeable for template matching which performance degrades rapidly as the size decreases. We learn that neural networks can achieve significantly better localization and classification performance, in particular convolutional networks with focus on high-resolution details such as those based on U-Net architecture.
Deeply Cascaded U-Net for Multi-Task Image Processing
Arxiv
Link: arxiv.org/abs/2005.00225
multi-task learning computer vision
In current practice, many image processing tasks are done sequentially (eg denoising, dehazing, followed by semantic segmentation). In this paper, we propose a novel multi-task neural network architecture designed for combining sequential image processing tasks. We extend U-Net by additional decoding pathways for each individual task, and explore deep cascading of outputs and connectivity from one pathway to another. We demonstrate effectiveness of the proposed approach on denoising and semantic segmentation, as well as on progressive coarse-to-fine semantic segmentation, and achieve better performance than multiple individual or jointly-trained networks, with lower number of trainable parameters.
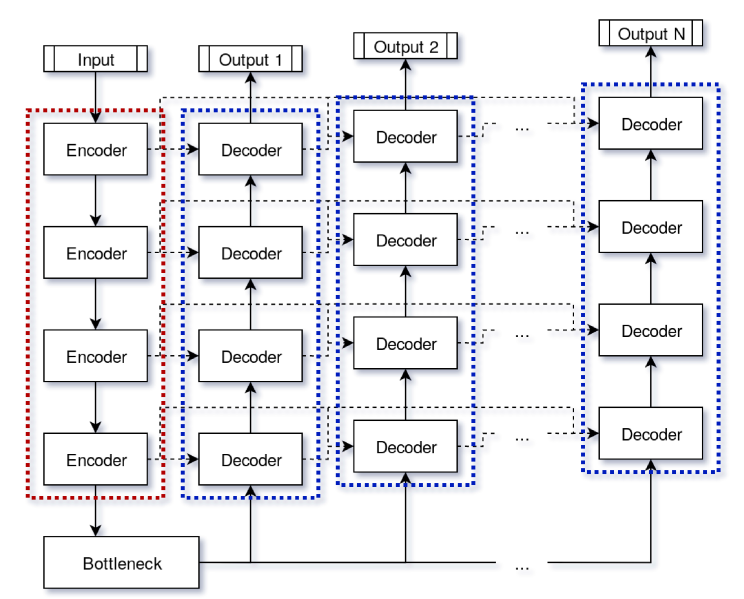
2019
SHREC’19 Track: Classification in Cryo-Electron Tomograms
3D Object Retrieval Workshop 2019, Leiden NL
Link: doi.org/10.2312/3dor.20191061
cryo-electron tomography simulator classification benchmark
Different imaging techniques allow us to study the organization of life at different scales. Cryo-electron tomography (cryo-ET) has the ability to three-dimensionally visualize the cellular architecture as well as the structural details of macro-molecular assemblies under near-native conditions. Due to beam sensitivity of biological samples, an inidividual tomogram has a maximal resolution of 5 nanometers. By averaging volumes, each depicting copies of the same type of a molecule, resolutions beyond 4Å have been achieved. Key in this process is the ability to localize and classify the components of interest, which is challenging due to the low signal-to-noise ratio. Innovation in computational methods remains key to mine biological information from the tomograms. To promote such innovation, we organize this SHREC track and provide a simulated dataset with the goal of establishing abenchmark in localization and classification of biological particles in cryo-electron tomograms. The publicly available dataset contains ten reconstructed tomograms obtained from a simulated cell-like volume. Each volume contains twelve different types of proteins, varying in size and structure. Participants had access to 9 out of 10 of the cell-like ground-truth volumes for learning-based methods, and had to predict protein class and location in the test tomogram. Five groups submitted eight sets of results, using seven different methods. While our sample size gives only an anecdotal overview of current approaches in cryo-ET classification, we believe it shows trends and highlights interesting future work areas. The results show that learning-based approaches is the current trend in cryo-ET classification research and specifically end-to-end 3D learning-based approaches achieve the best performance.
Automatic Generation and Stylization of 3D Facial Rigs
IEEE VR 2019, Osaka JP
Link: doi.org/10.1109/VR.2019.8798208
facial acquisition deformation transfer 3D reconstruction facial animation avatars
In this paper, we present a fully automatic pipeline for generating and stylizing high geometric and textural quality facial rigs. They are automatically rigged with facial blendshapes for animation, and can be used across platforms for applications including virtual reality, augmented reality, remote collaboration, gaming and more. From a set of input facial photos, our approach is to be able to create a photorealistic, fully rigged character in less than seven minutes. The facial mesh reconstruction is based on state-of-the art photogrammetry approaches. Automatic landmarking coupled with ICP registration with regularization provide direct correspondence and registration from a given generic mesh to the acquired facial mesh. Then, using deformation transfer, existing blendshapes are transferred from the generic to the reconstructed facial mesh. The reconstructed face is then fit to the full body generic mesh. Extra geometry such as jaws, teeth and nostrils are retargeted and transferred to the character. An automatic iris color extraction algorithm is performed to colorize a separate eye texture, animated with dynamic UVs. Finally, an extra step applies a style to the photorealis-tic face to enable blending of personalized facial features into any other character. The user's face can then be adapted to any human or non-human generic mesh. A pilot user study was performed to evaluate the utility of our approach. Up to 65% of the participants were successfully able to discern the presence of one's unique facial features when the style was not too far from a humanoid shape.
2017
Automated Generation of Animated 3D Facial Meshes: A Photogrammetry and Deformation Transfer-Based Model
MSc thesis, Utrecht University
Link: dspace.library.uu.nl/handle/1874/353287
facial acquisition deformation transfer photogrammetry facial animation
VR's recent, long expected breakthrough to the consumer market has enabled industry to deliver highly immersive experiences to consumers. However, creating photorealistic assets for such experiences is time consuming and requires a high level of expertise. To provide a remedy for this burden, this thesis presents a fully automated and validated model for the generation of animated 3D facial meshes, using photogrammetry and deformation transfer. Using a set of multi-camera photographs of a neutral face, we acquire facial geometry and appearance. Subsequent landmarking and ICP registration provide direct correspondence of the acquired facial mesh and an existing template. Then, using deformation transfer, we transfer existing blendshapes from template source to the obtained facial mesh. To assess the pipeline's effectiveness, we have conducted a set of user experiments. The results show that the automatically produced facial meshes faithfully represent subjects and can convey highly believable emotions. Compared to manual modeling and animation, the pipeline is more than 100x faster and produces results compatible with industry standard game engines, without any manual post-processing. As such, this thesis introduces a unique, fully automated, photogrammetry and deformation transfer-induced model for the generation of animated 3D facial meshes. On the one hand, this model can be expected to have both significant commercial and scientific impact. On the other hand, the model allows sufficient space for future improvement and tailoring.